Working paper
GGMncv: Nonconvex penalized Gaussian graphical models in R
2020
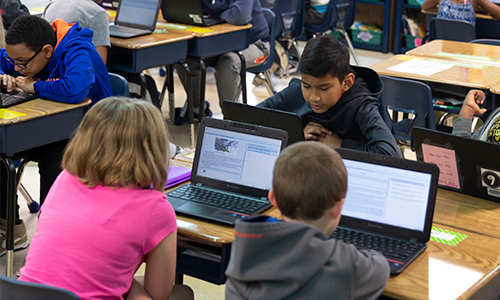
Description
Studying complex relations in multivariate datasets is a common task across the sciences. Recently, the Gaussian graphical model has emerged as an increasingly popular model for characterizing the conditional dependence structure of random variables. Although the graphical lasso (ā1-regularization) is the most well-known estimator, it has several drawbacks that make it less than ideal for model selection. There are now alternative forms of regularization that were developed specifically to overcome issues inherent to the `1-penalty. To date, however, these alternatives have been slow to work their way into software for research workers. To address this dearth of software, I developed the package GGMncv that includes a variety of nonconvex penalties, two algorithms for their estimation, plotting capabilities, and an approach for making statistical inference. As an added bonus, GGMncv can be used for nonconvex penalized least squares. After describing the various nonconvex penalties, the functionality of GGMncv is demonstrated through examples using a dataset from personality psychology.
See MoreTopics: Measurement & scaling